Introduction
In today’s digital landscape, data governance has emerged as a critical framework for organizations to ensure data integrity, privacy, and compliance. However, the arrival of generative AI presents new challenges and opportunities for data governance practices. As organizations harness the power of AI-generated content, they must navigate the complexities of maintaining data governance standards while leveraging the potential of these innovative technologies.
Generative AI, part of artificial intelligence, has gained fame for its ability to create synthetic data, images, text, and even entire narratives. Powered by deep learning algorithms, generative AI models analyze vast datasets to generate new content that mimics the patterns and styles of the input data. While this holds tremendous promise for various applications, including content creation, personalization, and simulation, it also raises concerns regarding data governance, ethics, and accountability.
At the forefront of these discussions is Amazon Web Services (AWS), a leading provider of cloud computing services. In a recent blog post AWS delves into the evolving landscape of data governance in the context of AI-driven content generation. The post explores the intersection of data governance principles and the deployment of generative AI models, offering insights and best practices for organizations navigating this complex terrain.
AWS emphasizes the importance of understanding the source of data used to train generative AI models and the potential biases and limitations inherent in these datasets. By adopting a proactive approach to data governance, organizations can mitigate the risks of unintended consequences and ensure that AI-generated content aligns with ethical standards and regulatory requirements.
Furthermore, AWS underscores the significance of continuous monitoring and auditing mechanisms to track the performance and behaviour of generative AI models over time. Organizations can identify and address emerging issues such as algorithmic biases, data drift, and model degradation through comprehensive data governance processes. Organizations can enhance accountability and maintain trust in AI-driven systems by integrating data governance into the AI development lifecycle.
In addition to technical considerations, AWS emphasizes the importance of fostering a culture of responsible AI within organizations. This requires promoting awareness, education, and collaboration among stakeholders to uphold ethical standards and address societal concerns related to AI technologies. By engaging in open dialogue and interdisciplinary collaboration, organizations can navigate AI-driven content generation’s ethical and regulatory complexities while fostering innovation and trust in AI technologies.
Key Challenges
One of the key challenges in implementing data governance for generative AI lies in balancing innovation with risk management. While generative AI offers unprecedented opportunities for creativity and efficiency, it also introduces novel data privacy, security, and misinformation risks. AWS advises organizations to adopt a risk-based approach to data governance, where risk assessments inform decision-making processes regarding developing, deploying, and monitoring AI models.
Moreover, AWS highlights the role of technology solutions in supporting data governance efforts in the age of generative AI. AWS offers a suite of AI services and tools designed to facilitate responsible AI development and deployment, including model explainability, fairness detection, and data lineage tracking. By leveraging these technologies, organizations can enhance transparency, interpretability, and accountability in AI-driven systems while complying with regulatory requirements and ethical standards.
As organizations navigate the complexities of data governance in the era of generative AI, collaboration and knowledge sharing play a crucial role in driving industry-wide best practices and standards. AWS encourages organizations to participate in forums, consortia, and working groups focused on AI ethics, data governance, and responsible AI development. By collaborating with peers, researchers, and policymakers, organizations can collectively address emerging challenges and shape the future of AI responsibly and ethically.
Drive Business Growth with AWS's Machine Learning Solutions
- Scalable
- Cost-effective
- User-friendly
Conclusion
Data governance in the age of generative AI requires a holistic approach encompassing technical, organizational, and societal dimensions. Organizations can harness the transformative potential of generative AI while mitigating risks and upholding societal trust by prioritizing transparency, accountability, and ethical use of data. AWS’s insights and best practices offer valuable guidance for organizations navigating this complex and dynamic landscape, ensuring that AI-driven innovation aligns with ethical principles and regulatory requirements.
Empowering organizations to become ‘data driven’ enterprises with our Cloud experts.
- Reduced infrastructure costs
- Timely data-driven decisions
FAQs
1. How can organizations leverage technology solutions to support data governance efforts in the era of generative AI?
ANS: – In the era of generative AI, technology solutions play a vital role in supporting data governance efforts. AWS offers a suite of AI services and tools designed to facilitate responsible AI development and deployment. These include model explainability, fairness detection, and data lineage tracking. By leveraging these technologies, organizations can enhance transparency, interpretability, and accountability in AI-driven systems while complying with regulatory requirements and ethical standards. Additionally, continuous monitoring and auditing mechanisms are essential for tracking the performance and behavior of generative AI models over time, allowing organizations to identify and address emerging issues such as algorithmic biases, data drift, and model degradation. Integrating data governance into the AI development lifecycle enhances accountability and maintains trust in AI-driven systems.
2. What are the primary challenges organizations face in implementing data governance for generative AI?
ANS: – Implementing data governance for generative AI presents several challenges for organizations. One key challenge lies in striking a balance between innovation and risk management. While generative AI offers exciting opportunities for creativity and efficiency, it also introduces novel risks related to data privacy, security, and misinformation. Organizations must adopt a risk-based approach to data governance, where thorough risk assessments inform decision-making processes throughout the development, deployment, and monitoring phases of AI models.
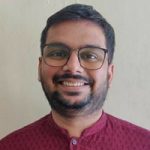
WRITTEN BY Nisarg Desai
Nisarg Desai is a certified Lead Full Stack Developer and is heading the Consulting- Development vertical at CloudThat. With over 5 years of industry experience, Nisarg has led many successful development projects for both internal and external clients. He has led the team for development of Intelligent Quarterly Remuneration System (iQRS), Intelligent Training Execution and Analytics System (iTEAs), and Cloud Cleaner projects among many others.
Comments