Voiced by Amazon Polly |
Introduction
Retrieval Augmented Generation (RAG) has emerged as a powerful technique to enhance the capabilities of large language models (LLMs). By combining the strengths of information retrieval and generative models, RAG systems can provide more accurate, informative, and relevant responses to user queries. Google Cloud Platform’s Vertex AI offers a comprehensive suite of tools and services to build and deploy RAG solutions efficiently.
Ready to lead the future? Start your AI/ML journey today!
- In- depth knowledge and skill training
- Hands on labs
- Industry use cases
Understanding RAG
RAG involves two primary steps:
- Retrieval: The system retrieves relevant information from a vast corpus of text or documents based on the user’s query.
- Generation: The retrieved information is used to generate a comprehensive and informative response using a generative language model.
The Role of Vertex AI
Vertex AI provides a robust platform for building RAG solutions, offering the following key benefits:
- Scalability: Handle large-scale datasets and complex queries efficiently.
- Flexibility: Customize your RAG system to meet specific requirements.
- Integration: Seamlessly integrate RAG into your applications.
- MLOps: Manage the entire machine learning lifecycle, from development to deployment.
Building a RAG Solution with Vertex AI
Here is a step-by-step guide to building a RAG solution using Vertex AI:
- Data Preparation:
- Corpus Creation: Assemble a comprehensive corpus of relevant text or documents.
- Vectorization: Convert the text into numerical representations (vectors) that can be used for similarity searches.
- Retrieval Component:
- Semantic Search: Use Vertex AI’s semantic search capabilities to efficiently retrieve relevant documents based on the user’s query.
- Indexing: Create indexes for your corpus to optimize search performance.
- Similarity Metrics: Choose appropriate similarity metrics (e.g., cosine similarity, Euclidean distance) to measure the relevance of retrieved documents.
- Generative Model:
- Selection: Select a suitable generative language model, such as BERT, GPT-3, or custom models.
- Fine-tuning: Fine-tune the model on your specific dataset to improve its performance on RAG tasks.
- Integration:
- Pipeline Creation: Create a pipeline that combines the retrieval and generation components.
- Query Handling: Implement a mechanism to handle user queries and trigger the RAG pipeline.
- Response Generation: Generate responses based on the retrieved information and the generative model.
- Deployment and Monitoring:
- Deployment: Deploy your RAG solution as a REST API or integrate it into your applications.
- Monitoring: Continuously monitor the performance of your RAG system and make necessary adjustments.
Key Considerations:
- Corpus Quality: Ensure the quality and relevance of your corpus to improve retrieval accuracy.
- Retrieval Efficiency: Optimize your retrieval system for speed and accuracy.
- Model Selection: Choose a generative model that aligns with your specific requirements and computational resources.
- Evaluation: Evaluate the performance of your RAG system using appropriate metrics (e.g., accuracy, relevance).
- Ethical Considerations: Address ethical concerns related to the use of RAG, such as bias and misinformation.
Advanced Techniques:
- Hybrid RAG: Combine multiple retrieval techniques (e.g., keyword-based, semantic) for improved performance.
- Contextual Retrieval: Consider the context of the user’s query to retrieve more relevant information.
- Knowledge Graph Integration: Incorporate knowledge graphs to enhance understanding and reasoning capabilities.
Conclusion
Vertex AI provides a powerful platform for building and deploying RAG solutions. By following the steps outlined in this guide and considering the key factors, you can create effective RAG systems that deliver valuable insights and enhance user experiences.
Stand out from the competition. Upskill with Google Cloud Certifications.
- Certified Instructors
- Real-world Projects
About CloudThat
CloudThat is a leading provider of Cloud Training and Consulting services with a global presence in India, the USA, Asia, Europe, and Africa. Specializing in AWS, Microsoft Azure, GCP, VMware, Databricks, and more, the company serves mid-market and enterprise clients, offering comprehensive expertise in Cloud Migration, Data Platforms, DevOps, IoT, AI/ML, and more.
CloudThat is the first Indian Company to win the prestigious Microsoft Partner 2024 Award and is recognized as a top-tier partner with AWS and Microsoft, including the prestigious ‘Think Big’ partner award from AWS and the Microsoft Superstars FY 2023 award in Asia & India. Having trained 650k+ professionals in 500+ cloud certifications and completed 300+ consulting projects globally, CloudThat is an official AWS Advanced Consulting Partner, Microsoft Gold Partner, AWS Training Partner, AWS Migration Partner, AWS Data and Analytics Partner, AWS DevOps Competency Partner, AWS GenAI Competency Partner, Amazon QuickSight Service Delivery Partner, Amazon EKS Service Delivery Partner, AWS Microsoft Workload Partners, Amazon EC2 Service Delivery Partner, Amazon ECS Service Delivery Partner, AWS Glue Service Delivery Partner, Amazon Redshift Service Delivery Partner, AWS Control Tower Service Delivery Partner, AWS WAF Service Delivery Partner and many more.
To get started, go through our Consultancy page and Managed Services Package, CloudThat’s offerings.
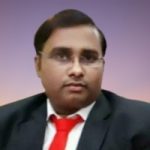
WRITTEN BY Abhishek Srivastava
Comments