Voiced by Amazon Polly |
Introduction
Generative AI, a subset of artificial intelligence focused on creating new data, has gained significant attention in recent years. From generating realistic images to crafting human-like text, its applications are vast and diverse. However, alongside its impressive capabilities, generative AI presents significant data privacy challenges. This blog explores these challenges and discusses ways to navigate them effectively.
Ready to lead the future? Start your AI/ML journey today!
- In- depth knowledge and skill training
- Hands on labs
- Industry use cases
Understanding Generative AI
Generative AI models, such as Generative Adversarial Networks (GANs) and Variational Autoencoders (VAEs), are designed to generate new content. These models learn patterns from large datasets and use this knowledge to create new, similar data. For instance, GANs can generate realistic images by learning from a dataset of photos, while models like OpenAI’s GPT-4 can write coherent and contextually appropriate text.
The Privacy Paradox
While generative AI holds great promise, it also raises significant privacy concerns. The primary issue lies in the training data. These models require vast amounts of data, often collected from various sources, including personal data. This necessity for large datasets creates a privacy paradox: the more data available to the AI, the better it performs, but this also increases the risk of privacy violations.
Data Collection and Consent
One of the primary concerns is the manner in which data is collected. Often, personal data is scraped from the internet without the knowledge or consent of the individuals involved. This practice can lead to the inclusion of sensitive information in the training datasets, posing a risk to individual privacy.
To address this, organizations must implement strict data collection practices. This includes obtaining explicit consent from individuals whose data is being used and ensuring transparency about how the data will be utilized. Additionally, anonymizing data can help mitigate privacy risks, although it is not a foolproof solution.
Data Security
The security of the data used to train generative AI models is another critical concern. If this data is not adequately protected, it can be vulnerable to breaches and misuse. Organizations must invest in robust security measures to safeguard the data, including encryption, secure storage solutions, and regular security audits.
Data Reconstruction
Generative AI models can inadvertently reveal sensitive information through data reconstruction. For example, a model trained on personal health records could potentially generate data that resembles the original records, compromising individual privacy. This issue highlights the need for careful management and monitoring of generative AI systems to prevent unintended data leakage.
Legal and Ethical Considerations
Navigating the challenges of generative AI and data privacy also involves understanding and complying with relevant legal and ethical standards. Various regulations, such as the General Data Protection Regulation (GDPR) in Europe and the California Consumer Privacy Act (CCPA) in the United States, provide guidelines on data privacy and protection.
GDPR and Generative AI
The GDPR sets stringent requirements for data collection, processing, and storage. Organizations using generative AI must ensure they comply with these regulations, particularly regarding obtaining consent and protecting personal data. Non-compliance can result in severe penalties and damage to an organization’s reputation.
Ethical AI Practices
Beyond legal compliance, ethical considerations are paramount. Organizations must adopt ethical AI practices, which include fairness, accountability, and transparency. This means developing AI systems that do not discriminate, are accountable for their outputs, and are transparent in their operations. Ethical AI practices help build trust and ensure the responsible use of generative AI.
Techniques to Mitigate Privacy Risks
To navigate the privacy challenges posed by generative AI, several techniques and best practices can be employed:
- Differential Privacy
Differential privacy is a technique that adds noise to the data, making it difficult to identify individual records. This approach allows organizations to use large datasets while preserving individual privacy. By ensuring that the output of generative AI models does not reveal specific data points, differential privacy can help mitigate privacy risks.
- Federated Learning
Federated learning is another promising technique where the model is trained across multiple decentralized devices or servers holding local data samples, without exchanging them. This method enhances privacy by keeping the data on local devices and only sharing model updates. Federated learning can be particularly useful in sensitive areas such as healthcare and finance.
- Model Auditing and Monitoring
Regular auditing and monitoring of generative AI models are essential to identify and address privacy risks. This involves analyzing the model’s outputs to ensure they do not inadvertently reveal sensitive information. Continuous monitoring helps in maintaining the integrity and privacy of the data used.
The Path Forward
Generative AI holds immense potential, but its development and deployment must be handled with care to address data privacy challenges. By adopting robust data collection and security practices, complying with legal and ethical standards, and leveraging privacy-preserving techniques, organizations can navigate these challenges effectively.
As we move forward, a collaborative approach involving researchers, policymakers, and industry stakeholders is crucial. Together, we can ensure that the benefits of generative AI are realized while safeguarding individual privacy. Balancing innovation with privacy will pave the way for a future where generative AI can thrive responsibly and ethically.
Enhance Your Productivity with Microsoft Copilot
- Effortless Integration
- AI-Powered Assistance
About CloudThat
CloudThat is a leading provider of Cloud Training and Consulting services with a global presence in India, the USA, Asia, Europe, and Africa. Specializing in AWS, Microsoft Azure, GCP, VMware, Databricks, and more, the company serves mid-market and enterprise clients, offering comprehensive expertise in Cloud Migration, Data Platforms, DevOps, IoT, AI/ML, and more.
CloudThat is the first Indian Company to win the prestigious Microsoft Partner 2024 Award and is recognized as a top-tier partner with AWS and Microsoft, including the prestigious ‘Think Big’ partner award from AWS and the Microsoft Superstars FY 2023 award in Asia & India. Having trained 650k+ professionals in 500+ cloud certifications and completed 300+ consulting projects globally, CloudThat is an official AWS Advanced Consulting Partner, Microsoft Gold Partner, AWS Training Partner, AWS Migration Partner, AWS Data and Analytics Partner, AWS DevOps Competency Partner, AWS GenAI Competency Partner, Amazon QuickSight Service Delivery Partner, Amazon EKS Service Delivery Partner, AWS Microsoft Workload Partners, Amazon EC2 Service Delivery Partner, Amazon ECS Service Delivery Partner, AWS Glue Service Delivery Partner, Amazon Redshift Service Delivery Partner, AWS Control Tower Service Delivery Partner, AWS WAF Service Delivery Partner and many more.
To get started, go through our Consultancy page and Managed Services Package, CloudThat’s offerings.
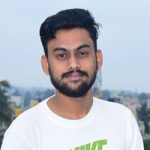
WRITTEN BY Keerthish N
Comments