Voiced by Amazon Polly |
Introduction
Evaluating foundation models is essential to ensure their reliability, effectiveness, and alignment with business goals. As generative AI becomes central to applications across industries, ranging from chatbots and content generation to data summarization and customer service, assessing the output of these models becomes increasingly critical.
Amazon Bedrock, a fully managed service to build and scale generative AI applications, simplifies this process by offering built-in model evaluation capabilities. Whether you’re testing Anthropic Claude, AI21 Labs’ Jurassic, Meta’s Llama, or Amazon Titan, Bedrock provides both automatic and human evaluation methods to help assess the model’s quality. This blog walks you through the evaluation process and how it empowers teams to make data-driven decisions.
Explore and Interpret Information in an Interactive Visual Environment
- No upfront cost
- Row level security
- Highly secure data encryption
Importance of Model Evaluation
Before deploying a foundation model into production, it’s important to assess its accuracy, coherence, and safety. Poorly performing models can lead to:
- Misinformation in generated outputs
- Inconsistent or irrelevant responses
- Biases that may affect fairness or inclusion
- Security or reputational risks if inappropriate content is produced
By evaluating a model early, organizations can uncover such issues before they impact users. For example, in a customer support use case, inaccurate responses could lead to customer dissatisfaction or even legal concerns. Proper evaluation also ensures that the model is fit for purpose, whether you’re using it for summarization, code generation, or question-answering.
Amazon Bedrock offers two robust approaches to model evaluation
Automatic Evaluation
Automatic evaluation in Bedrock enables users to assess model responses using pre-set metrics and datasets without requiring manual intervention. There are two key modes:
- Programmatic Evaluation
Programmatic evaluation allows users to test a model using input-output pairs. These pairs can be from a predefined dataset or a custom dataset. You define the metrics, such as BLEU (for text similarity), ROUGE (for summarization), or toxicity scores, and Bedrock automatically evaluates the model’s responses at scale. This is ideal for teams looking to test many prompts quickly and objectively.
Example: If you’re building a product description generator, you can input sample product titles and compare generated descriptions against reference descriptions using similarity scores.
- Model as a Judge
This method leverages another foundation model, like Claude or Titan, to assess the outputs of your primary model. You give it evaluation criteria (e.g., relevance, correctness, tone), and the evaluator model scores the outputs accordingly. This helps in evaluating nuanced aspects of a response that traditional metrics might miss.
Example: In a chatbot scenario, you can ask the model-as-judge to rate answers based on helpfulness, appropriateness, and empathy.
Human Evaluation
Even with the most advanced automation, human judgment remains essential in evaluating nuanced or subjective aspects of language. Bedrock supports two modes of human evaluation:
- AWS Managed Work Team
Amazon provides a curated team of human reviewers who evaluate up to two different models’ outputs. You define the evaluation rubric (e.g., factuality, fluency, tone), and reviewers assess which model performs better across prompts. This is especially useful when you’re deciding between two providers, like Claude vs. Titan.
Use Case: Comparing how two models summarize medical articles for accuracy and readability.
- Bring Your Own Work Team
If you want internal experts or business stakeholders to handle the evaluation, Bedrock enables you to assign the task to your own workforce. You can also define job-specific evaluation forms and standards, ensuring the reviewers focus on what matters most to your use case.
Use Case: A legal team evaluates generative model outputs to ensure contract summaries maintain key legal clauses and intent.
Reviewing and Analyzing Results
Evaluation is not complete without proper analysis. Amazon Bedrock automatically stores evaluation results in Amazon S3, allowing users to track and compare model performance over time.
Key Steps After Evaluation:
- Visualize Results: Use tools like Amazon QuickSight or SageMaker Studio to analyze performance metrics and evaluation feedback.
- Detect Issues: Spot patterns in bias, hallucination, or low coherence in model responses.
- Refine Your Prompts: Prompt engineering may improve results without switching models.
- Experiment with Models: If one model underperforms, switch to an alternative provider in Bedrock with minimal code changes.
- Create a Feedback Loop: Feed evaluation data back into your model improvement or prompt tuning process.
Tip: Track metrics like model score variance across use cases (e.g., customer service vs. technical support) to decide on specialization.
Conclusion
Amazon Bedrock provides a flexible, scalable, and comprehensive solution for evaluating generative AI models. Whether you’re running automated tests on hundreds of prompts or collecting insights from human reviewers, Bedrock’s evaluation tools help ensure your models are accurate, fair, and aligned with your business goals.
By integrating both quantitative metrics and qualitative human input, you can confidently select, fine-tune, and deploy foundation models that perform consistently across real-world applications. Understanding this evaluation process is essential to delivering generative AI applications that are not only powerful but also responsible and trustworthy.
References
Enhance Your Productivity with Microsoft Copilot
- Effortless Integration
- AI-Powered Assistance
About CloudThat
CloudThat is a leading provider of Cloud Training and Consulting services with a global presence in India, the USA, Asia, Europe, and Africa. Specializing in AWS, Microsoft Azure, GCP, VMware, Databricks, and more, the company serves mid-market and enterprise clients, offering comprehensive expertise in Cloud Migration, Data Platforms, DevOps, IoT, AI/ML, and more.
CloudThat is the first Indian Company to win the prestigious Microsoft Partner 2024 Award and is recognized as a top-tier partner with AWS and Microsoft, including the prestigious ‘Think Big’ partner award from AWS and the Microsoft Superstars FY 2023 award in Asia & India. Having trained 650k+ professionals in 500+ cloud certifications and completed 300+ consulting projects globally, CloudThat is an official AWS Advanced Consulting Partner, Microsoft Gold Partner, AWS Training Partner, AWS Migration Partner, AWS Data and Analytics Partner, AWS DevOps Competency Partner, AWS GenAI Competency Partner, Amazon QuickSight Service Delivery Partner, Amazon EKS Service Delivery Partner, AWS Microsoft Workload Partners, Amazon EC2 Service Delivery Partner, Amazon ECS Service Delivery Partner, AWS Glue Service Delivery Partner, Amazon Redshift Service Delivery Partner, AWS Control Tower Service Delivery Partner, AWS WAF Service Delivery Partner, Amazon CloudFront, Amazon OpenSearch, AWS DMS, AWS Systems Manager, Amazon RDS, and many more.
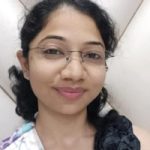
WRITTEN BY Nehal Verma
Comments